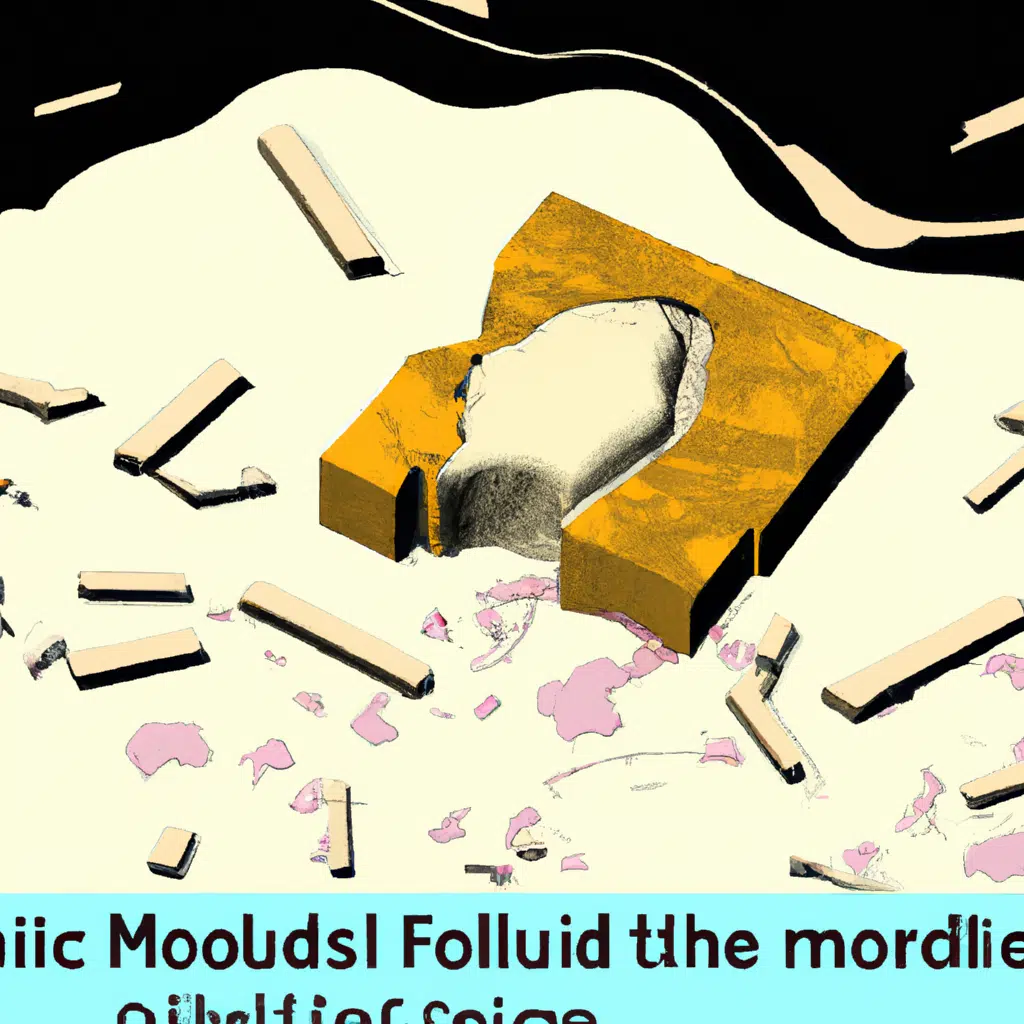
In recent years, the field of flood risk mapping has undergone a significant transformation thanks to the advent of innovative machine learning algorithms. These powerful algorithms have revolutionized the way we understand and predict flood risks, enabling us to better prepare for and mitigate the devastating consequences of flooding. In this article, we will explore the groundbreaking advancements in machine learning algorithms and their application in flood risk mapping. By harnessing the power of these algorithms, we can reshape the future of flood management and protect vulnerable communities from the threats posed by floods.
Understanding Flood Risk Mapping
Flood risk mapping is the process of assessing and predicting the likelihood and magnitude of flooding in a particular area. Traditionally, this has been done through empirical methods, which rely on historical data and physical models. While these methods have provided valuable insights, they often fail to capture the complex and dynamic nature of floods. This is where machine learning algorithms come into play.
The Role of Machine Learning Algorithms
Machine learning algorithms, a subset of artificial intelligence, are designed to learn from data and make predictions or decisions without being explicitly programmed. These algorithms can analyze large volumes of data, identify patterns, and make accurate predictions based on these patterns. In the context of flood risk mapping, machine learning algorithms can process vast amounts of data, including historical flood records, climate data, topographic information, and more, to create sophisticated models that can predict flood risks with unprecedented accuracy.
Advantages of Machine Learning Algorithms in Flood Risk Mapping
Machine learning algorithms offer several key advantages over traditional methods in flood risk mapping:
- Improved Accuracy: By analyzing complex data sets, machine learning algorithms can identify subtle patterns and relationships that traditional methods may overlook. This leads to more accurate flood risk predictions and better-informed decision-making.
- Real-Time Monitoring: Machine learning algorithms can continuously analyze incoming data, allowing for real-time monitoring of flood conditions. This enables authorities to take proactive measures and issue timely warnings to at-risk communities, potentially saving lives and reducing damages.
- Adaptability: Machine learning algorithms can adapt to changing environmental conditions and incorporate new data as it becomes available. This ensures that flood risk maps remain up-to-date and relevant, even in the face of climate change and other evolving factors.
Innovative Machine Learning Techniques in Flood Risk Mapping
Several innovative machine learning techniques have emerged in recent years, enhancing the accuracy and efficiency of flood risk mapping. Let’s explore some of these techniques:
1. Deep Learning
Deep learning is a subfield of machine learning that focuses on training artificial neural networks with multiple layers to recognize complex patterns and make predictions. In flood risk mapping, deep learning algorithms can analyze various data sources, such as satellite imagery, rainfall measurements, and river gauge data, to accurately predict flood risks at a high spatial resolution.
2. Ensemble Learning
Ensemble learning involves combining multiple machine learning models to improve prediction accuracy. In flood risk mapping, ensemble learning techniques can be used to merge predictions from different algorithms, leveraging their individual strengths and compensating for any weaknesses. This results in more robust and reliable flood risk assessments.
3. Transfer Learning
Transfer learning is a technique where knowledge gained from solving one problem is applied to a different but related problem. In the context of flood risk mapping, transfer learning allows us to leverage pre-trained models trained on similar environmental conditions and apply them to new locations with limited data. This significantly reduces the need for extensive data collection and model training, making flood risk mapping more accessible and cost-effective.
Case Studies: Successful Implementation of Machine Learning Algorithms
To showcase the effectiveness of machine learning algorithms in flood risk mapping, let’s examine a few notable case studies:
1. The Netherlands’ Deltares System
The Netherlands, a country prone to flooding, has implemented a state-of-the-art flood risk mapping system developed by Deltares. This system utilizes machine learning algorithms to analyze various data sources, including historical flood records, soil types, and water management infrastructure. By combining these data sets with advanced machine learning models, the system accurately predicts flood risks and assists in the development of effective flood management strategies.
2. SMART-FLOOD Project in Thailand
Thailand is another country that faces significant flood risks, particularly during the monsoon season. The SMART-FLOOD project, a collaboration between Thai and European researchers, applies machine learning algorithms to predict flood risks in urban areas. By analyzing satellite imagery, weather data, and historical flood records, the project has successfully provided timely flood warnings and improved urban planning to mitigate the impact of floods.
3. FloodSense Project in the United States
The FloodSense project, led by researchers at the University of California, Irvine, aims to improve flood risk mapping in urban environments using machine learning algorithms. By analyzing data from various sources, including social media, weather sensors, and historical flood records, the project can accurately predict flood risks in real-time. These predictions help emergency responders and city planners make informed decisions to protect vulnerable communities and reduce property damage.
Conclusion
In conclusion, innovative machine learning algorithms have revolutionized flood risk mapping, offering unprecedented accuracy, real-time monitoring, and adaptability. By harnessing the power of these algorithms, we can better understand and predict flood risks, enabling us to develop more effective flood management strategies and protect vulnerable communities. As we continue to advance in the field of machine learning, the future of flood risk mapping looks promising, providing hope for a safer and more resilient world.